Patterns in data in drinking water consumption can be used to save energy and to detect leakages, says Dr. Martijn Bakker in his PhD thesis.
Human behaviour is pretty predictable finds Dr. Martijn Bakker who did his PhD research at the department of Water Management at the faculty of Civil Engineering and Geo Sciences. His analysis of datasets covering six years of water demand in six different areas in the Netherlands (varying between 2,400 to 950,000 inhabitants) showed that water demand can be forecasted, pretty precisely, 24 hours (at 15 minute intervals) in advance. The mean error was between 1.5 – 5.1%. Adding weather information improved the forecasts slightly, because at warm evenings there is additional demand from people spraying their lawns.
Using historic data to forecast demand is done with the optimised control model (OCM) that is used by more than half of the water supply systems in the Netherlands. The OCM decreases variation in production flow by 75%, the turbidity level in water by 17% lower and the energy use with 5%. At a test in Poland, the optimised control model even allowed for 29% pump power reduction, resulting in 18% less leakage (because of the lower pressure) and more than 11% energy savings.
Demands significantly exceeding forecasts may be an indication for a leak or a pipe burst. If actual demand is more than 5% higher than the forecast, an alarm is issued. The result of a test on nine sets of historic pipe burst data is that 80-90% of the bursts could be detected within twenty minutes. The data model also produced false alarms on 3% of the days without incidents. Leakage detection sensitivity is a trade-off between maximal sensitivity and minimal false alarms. Incidentally, not all pipe bursts can be detected this way: small leakages may stay unnoticed for a long time resulting in considerable water losses.
In a sense, the analysis of historic data sets is just a warming-up. The revolution will really get going once smart water meters are generally deployed. Or, as Bakker says in one of his propositions: “Introduction of smart domestic water meters provides the opportunity to dramatically increase the sensor density in water distribution networks, which will induce a revolution in the field of on-line network monitoring and control.”
In the meantime, water companies may benefit from monitoring social media, says Bakker, since people will complain more easily on Facebook or Twitter about drinking water failures than contact their water provider.
Bakker (1970) graduated from TU Delft in 1995 on water demand forecasting and production flow control, after which he began working at DHV at the same subject. He returned in 2011 to the TU as a part-time PhD student.
–> Martijn Bakker, Optimised control and pipe burst detection by water demand forecasting, PhD supervisor Professor Luuk Rietveld (CEGS), 17 October 2014. Research funded by Dutch governmental Innowator programme.
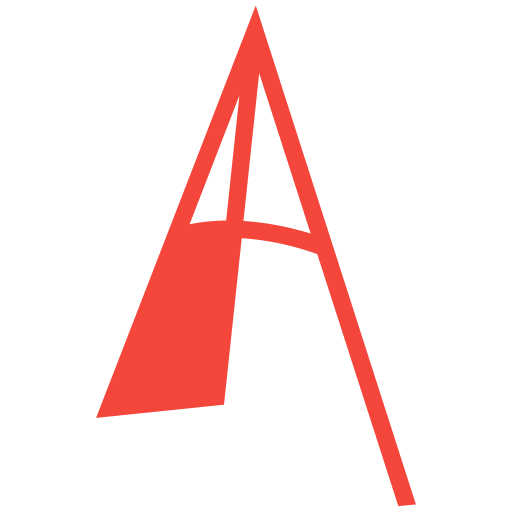
Comments are closed.