After the Dutch Government fell over the child benefit scandal last week, the question of meaningful human control over AI systems is now more urgent than ever.
AI systems watch for 'suspicious' behaviour in public spaces. (Photo: Pxhere)
Computer scientist and Master of Ethics and Technology Scott Robbins (1984) wrote his PhD thesis on machine learning & counterterrorism. He concludes that decisions which require an explanation (why was this man shot, why do you store my data, why are you accusing me of fraud) must not be delegated to AI powered machines. This fundamental insight on ‘meaningful human control’ reaches beyond the war on terror.
Why did you choose counterterrorism as your subject of study?
“I wanted a context where the goal is good. Countering terrorism is an unquestionably good goal. We don’t want terrorists to cause harm to innocent people. So, starting from this good goal I wanted to then try to understand under what conditions the state can use artificial intelligence to achieve that goal in a way that is both effective and ethical.”
You write that the use of machine learning in counterterrorism is increasing. Could you give me some examples of what we’re talking about?
“The most prominent example is autonomous weapon systems where weapon systems are able to shoot a target or to automatically fire on what the system perceives to be an enemy. A lot of this is driven by artificial intelligence in the background, such as facial recognition. A more benign example which is really employed quite extensively, is at borders or airports. If you cross into a new country or into the European Union, you’re likely to be subjected to a facial recognition system which checks you against the database of known or suspected terrorists. It seems to be part of a larger system of border control where you might also want to check if your face matches the photo on your passport – stuff like that. There is an interesting example in which AI is deployed to detect somebody acting suspiciously. Take the CCTV cameras at an airport. They have everything from detecting somebody leaving a bag, looking nervous or loitering in an area that they maybe shouldn’t be standing around in. These systems are making judgements all the time.”
What is the problem with these advanced surveillance systems?
“There is a huge number of problems with facial recognition. We know that facial recognition works quite well for white middle-aged males. But it works terribly for people of colour and women. Part of the reason is that the engineers in Silicon Valley have more access to faces and pictures of people that are white and middle-aged and male. And not as much of people who don’t look like them. These problems could be fixed or not. But what we’re noticing now is that in places where people are selected for further inspection at an airport, this is more likely to happen because the facial recognition system fails on them or misclassifies them. And then of course you get red-flagged and you have to go and get interviewed. And so, the issue is then: is a part of the population overly burdened, simply by virtue of their skin colour, rather than by virtue of their being suspicious?”
‘Neutral language would help’
What is the alternative?
“What I say is that decisions requiring judgement, meaning ethical decisions or aesthetic decisions – decisions about ethics or beauty – should not be delegated to machines, the reason being that we can’t judge their efficacy. To give an example that makes it clear: an algorithm watching someone through a camera sees that they’re loitering. That is one thing. That could mean we see the same person wandering around in this two metre environment for more than two minutes. That is very clear, we know that the system has worked or not worked, and we can make judgements based on that. Now, another system watches the same person and says: this person is suspicious. But ‘suspicious’ is a judgement. You can’t determine whether that person is suspicious or not, so you can never tell anything about the efficacy of the system.”
Is it all in the language then?
“Neutral language would help. Descriptive terms like ‘this person is loitering’ or ‘this person left their bag’ is neutral language. When we use the language correctly it’s not as bad. Of course, it’s still a security context, we’re taught to be on alert. But it’s much different from classifying someone as ‘suspicious’. The suspicious thing has multiple problems, right? Not only are people primed to be more negative towards that person, but we don’t know why the system flagged them as suspicious. It might be because of the colour of their skin, or the way their face looks – we don’t know. In the future we could find out if the system flags more people on the basis of their skin colour, or their race. And we’ve discovered that in numerous other contexts. Amazon for example was using AI to hire people and they had to quickly shut it down because they found the system was heavily biased towards giving higher level positions to people just because they were men, denying positions to people because they were women. The reason is fairly obvious: that the people that were in management positions at Amazon already were men. So the training data, the examples used, were all of men so of course the machine picked up on that, giving even more positions to men.”
‘Now we find they’re as bad as we are’
Isn’t it ironic that the machine reflects your own biases?
“Exactly. You know, part of the reason that people want to use machines is because machines are supposed to be neutral, objective and free of biases. Now we find they’re as bad as we are because they’re trained on our data from the past.”
Shouldn’t there be public control over the security systems that safeguard us?
“I think there should be some public control. In an ideal society, or in the European societies that we’re trying to realise, there is public control in some sense. We’re not in the room developing the system. There will have to be some confidentiality with regards to the systems intended to prevent serious crimes. If we were to make all this information public, everyone would know how it works and that’s not going to help counter terrorism.
However, there are some abstract goals and principles that we can set out in societies to say: we don’t know how it works but we can demand reasonable suspicion as a condition for data collection. Intelligence agencies have to be reasonably articulate why they think somebody deserves intrusive surveillance. That doesn’t say anything about AI, but what it does do is ensure that any systems used in this context – AI or not – will have to live up to that principle. We could demand that any version of AI should have a human being, a human intelligence analyst, behind it to articulate reasonable suspicion against a certain person before we start collecting all their information. It’s these principles that I think society and the public has a part in developing. And then it will be up to the people behind closed doors to decide how that works in practice. And hopefully with some government oversight to make sure that intelligence agencies are not overreaching, as they have been shown to do in the past.”
I see that you will be working with the Center for Advanced Security, Strategic and Integration Studies (CASSIS) at Bonn University. Will you continue the same line of work?
“Yes, it’s very much a continuation. I will definitely talk about meaningful human control, but in this next project I would like to push this idea further and really understand how humans can keep meaningful control over algorithms in a way that helps us realise our liberal democratic ideal of a society.”
- Scott Alan Robbins, Machine Learning & Counter-Terrorism, Ethics, Efficacy, and Meaningful Human Control, PhD defence 22 February 2021, Promotors: Prof. Seumas Miller and Prof. Ibo van der Poel.
- For more on Scott Robbins, visit his website and discover why AI should be boring.
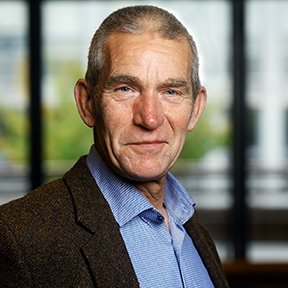
Heb je een vraag of opmerking over dit artikel?
j.w.wassink@tudelft.nl
Comments are closed.