For the elderly, people with regional accents and non-native speakers, speech recognition systems are a pain. They don’t catch your meaning and are biased, TU research shows.
Speech recognition algorithms come at a cost. They are discriminatory. (Photo: Hannah Wei/Unsplash)
We are constantly confronted with them; whether we call our insurance company, telecom provider or municipality, chances are that we first have to converse with a computer before being put through to an operator of flesh and blood.
Companies use automatic speech recognition (ASR) algorithms to save on telephone operators and thus money. But these algorithms come at another cost. They are discriminatory. Or so researchers from TU Delft, the University of Amsterdam and the Netherlands Cancer Institute write in their study entitled Quantifying Bias in Automatic Speech Recognition which was recently published on arxiv.org.
‘These are troubling ethical issues’
“Because of the relevance that spoken language plays in our lives, it is so important that ASR systems deal with the variability in the way people speak,” says Olya Kudina, Assistant Professor at the Ethics and Philosophy of Technology Department (Faculty of Technology, Policy and Management) and one of the authors. “The bias in the datasets makes some people more visible than others and some ways of speaking seemingly more relevant than others. These are troubling ethical issues.”
“ASR plays an increasingly important role in our lives”, adds her colleague and co-author Odette Scharenborg, expert in speech recognition technology at the Faculty of Electrical Engineering, Mathematics & Computer Science. “They can enable older people to live independently at home for longer as more and more devices that work with voice control are introduced. And they can unlock the world for people who are low-literate or illiterate or for people who cannot type due to muscle diseases.”
State-of-the-art ASR systems are based on deep neural networks (DNNs). DNNs are often considered to be a harbour of objectivity because they follow a clear path against the set parameters of the dataset, the researchers write in their article. But evidence suggests that even state-of-the-art ASRs struggle with the large variation in speech, variation due to things like gender, age, speech impairment, race, and accents.
Perpetuating a racial divide
Recent studies from the US amongst others have shown troubling evidence that voice assistants may perpetuate a racial divide by misrecognising the speech of black speakers more often than of white speakers. Speech impairments are also known to cause many problems. Take for instance impairments related to dysarthria, strokes, oral cancer or cleft lip and palate.
And ASR systems are typically trained using speech from native speakers of a ‘standard’ variant of that language, inadvertently discriminating not only the speech of non-native speakers through high error rates but also that of speakers of regional or sociolinguistic variants of the language.
How bad is the situation for Dutch ASR systems? To find out, the researchers began by feeding an ASR system sample data from The Spoken Dutch Corpus (Corpus Gesproken Nederlands), a project to construct a database of contemporary standard Dutch as spoken by adults in The Netherlands and Flanders. It covers speaking styles and includes broadcast news and telephone conversations. The researchers used about 400 hours of training material spoken by men and women aged 18 to 65. They also worked with data from senior citizens, children and non-native speakers in the Netherlands with a wide range of native languages, including Turkish and Moroccan Arabic. And they used data from Flanders.
Struggling with Flemish
Their experiments show that the ASR recognised female speech more reliably than male speech. The system also struggled to recognise speech from older people compared with younger people, potentially because the former group articulated less clearly than the younger speakers. And it had an easier time recognising speech from native speakers versus non-native speakers, irrespective of age. For native Dutch speakers, the speech from Flanders obtained the worst ASR performance.
Developers of ASR systems should diversify the data sets they use to train the algorithm much more, the scientists conclude. They should be ‘aiming for a balanced representation of all types of speakers in the dataset’.
Scharenborg adds that the challenges she and her colleagues face are huge. “The variation is just tremendous, but the stakes are high. Everyone should be able to use speech recognition. We should therefore also be looking at smarter ways in which deep neural networks use the data and develop new AI architectures. I coined the term ‘inclusive automatic speech recognition’. This field of research is still in its infancy.”
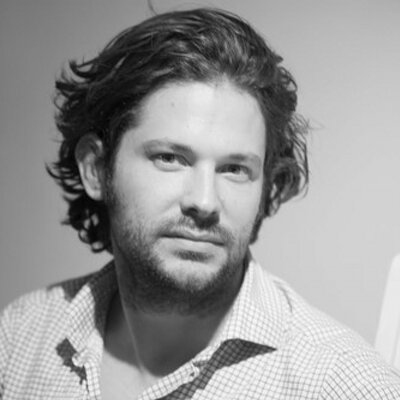
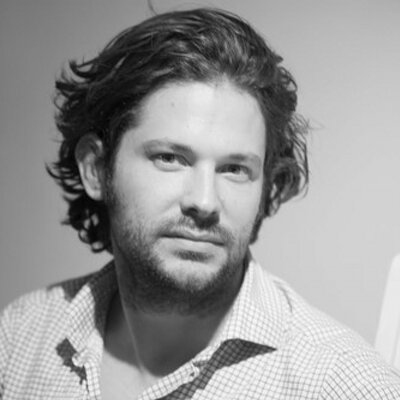
Do you have a question or comment about this article?
tomas.vandijk@tudelft.nl
Comments are closed.